A recent study challenges previous ideas of how technology affects employment
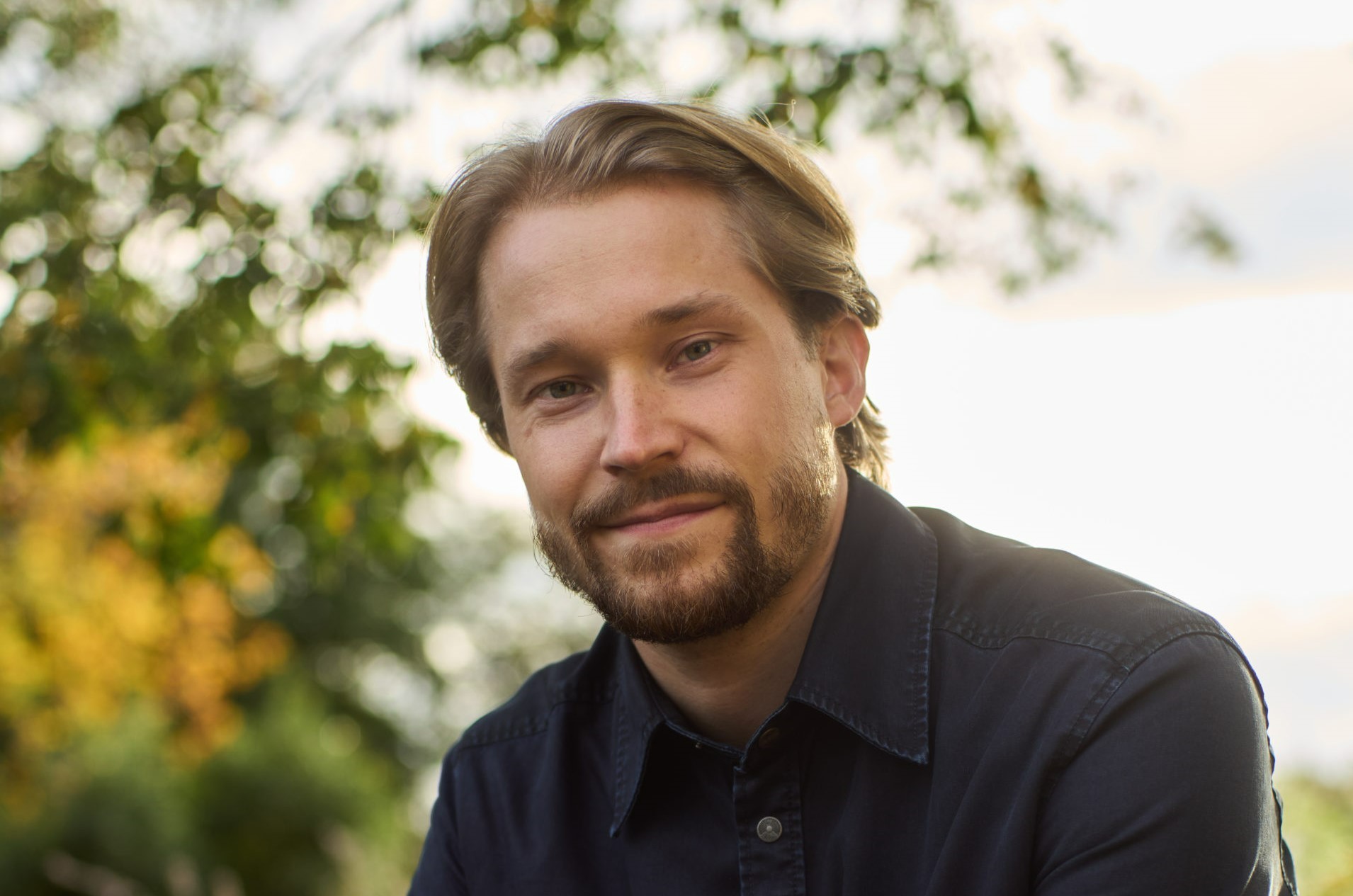
Summary
- The impact of new technologies – particularly on industrial work – has been studied at length, often from the perspective of displacement, that is, on the assumption that technology will replace certain routine jobs and give way to new, more demanding jobs.
- A recent study measured specific impacts at the company level and concluded that rather than destroying jobs, new technologies actually result in employment growth. This is in contrast with previous thinking of how technology affects jobs.
- Joonas Tuhkuri (PhD, MIT) is an economist and Assistant Professor of Economics at Stockholm University from 2023. He lives in Berlin. In his research he uses a large set of novel data to study technology, work, skills and personalities. In the interview below, Joonas shares some insights into his recently published study ‘New Evidence on the Effect of Technology on Employment and Skill Demand’ and answers questions about the future of work.
You are one of the co-authors of a recent study conducted by MIT, Etla Economic Research and the Labour Institute for Economic Research (Labore) on the impacts of technology on work and skills demand. Can you tell us more about that?
We set off to answer a familiar question: How will technology affect employment and skill demand, particularly in the industrial sector? We addressed the question from two perspectives: firstly, what is the impact of technologies on the number of jobs, and secondly, what is their impact on the nature of jobs?
A common assumption is that technology will displace certain routine tasks and give way to more complex and demanding ones. This is partly true. Another common assumption is that more demanding tasks require higher education. The skill-biased technological change hypothesis suggests that if an industrial company invested in robots or CNC machines, that would result in employee turnover, and thereby drive income inequality in Western countries.
It was precisely this growing income gap, which is particularly notable in the United States and the United Kingdom, that sparked our research. The impacts of technology have not been previously measured satisfactorily at a single company level, nor has the chain of effects been studied in detail. Instead, the focus has been on bigger trends. I wanted to measure a company’s investments in machinery or technology and its range of employees in detail and with precision.
This is an area that doesn’t really lend itself to research, largely because companies that do invest in technology are very different from those that don’t. To make results comparable, we wanted to create an experimental design in which a company investing in technology was fairly similar to one that didn’t. We were able to engineer such a design by using the business subsidies granted by the Centres for Economic Development, Transport and the Environment, or ELY Centres. All the companies included had applied for business subsidies from ELY Centres, but for eligibility reasons some companies were granted technology support while others were not.
Your results showed that instead of destroying jobs, new technologies actually led to an increase in employment. So, robots are not stealing our jobs after all?
The short answer is no. This finding was something of a surprise to us.
Our results showed that employment grew significantly in subsidised companies in comparison to non-subsidised companies, but there were no changes in the employee or skill composition. This suggests that it is highly unlikely that robots would displace workers.
For the purpose of our study, we excluded all other investments except those made in robots, and it was very clear that the number of employees in these companies did not decrease – in fact, quite the opposite. Similarly, employees with secondary education were not displaced; instead, demand for those workers was growing. This finding stands in stark contrast to the general view among economists.
How do you explain this?
It’s quite simple. The companies we studied are highly specialised manufacturers of products with high added value. This description applies to most industrial enterprises. While cost cuts may be on their agenda, they make investments for entirely different reasons. Lift and crane manufacturers, for instance, want to make better products and thereby grow their market share and win new customers. Replacing employees with machines would not serve their purpose.
Your study involved Finnish industrial companies. Would you say your findings can be generalised globally?
Research in this field with this accuracy is scant, making it difficult to offer any definitive answers. The way I see it, there are two possibilities: Either our findings are different because we were able to conduct precise measurements in the Finnish context. Following this logic, if we conducted another study with equal precision elsewhere, the results would be similar. Or the results may be different because the sector of industry we studied was dissimilar to others.
Most likely it is a both-and situation. Mass production is no longer the standard business model; instead, industrial companies are increasingly focusing on high-quality products with high added value. In the high value-added industries, the direct cost benefits achieved through automation have potentially already been largely utilized. But mass production is still alive and well in developing countries and in some parts of the United States.
It is a distinct possibility that our results may be globally generalisable in the type of industry that we studied, but not in industrial sectors producing low added value goods such as sugar or pulp.
What are the broader implications on societies and on employment policy planning?
One of the much debated and controversial issues is the introduction of a robot tax. There has been much discussion on whether to tax robots because they are harmful for workers, but our research findings suggest it would not necessarily be a plausible idea, at least from the worker protection perspective. On the contrary, our research indicates that workers are better off in companies that invest in robots.
The support provided by ELY Centres in Finland constitutes what you might call a reverse robot tax, but it seems to have a positive impact on employment. Although we have not carried out a formal cost-benefit analysis, I think it’s safe to say that this type of research will provide legitimate policy options with a concrete price and impact predictability.
As a part of your doctoral dissertation you studied the impact of manufacturing decline on children and young people in the United States. What were your conclusions?
Unlike most studies that focus on the adaptation and retraining of middle-aged employees, I wanted to address what I considered a much bigger issue: what happens to children in these left-behind places. This approach offers a longer-term perspective on the future of work, as opposed to the traditional scenario with a 10–15-year horizon.
I compared locations that had lost manufacturing jobs with similar locations with no job losses. My research design was based on changes in China’s trading patterns in the early 2000s. When China opened up its trade, manufacturing jobs disappeared in the US, but in some locations more than others.
Surprisingly, my research showed that manufacturing decline led to a decrease in high school dropout rates and increased access to further education, especially tertiary education. This makes perfect sense from the economic theory perspective: young people responded to the absence of low education jobs by seeking a higher level of education. There was demand, or the desire to gain an education, but whether this resulted in access to education depended on the availability of education services.
Loss of manufacturing jobs is often brought up, but what about office jobs? Will they be affected by new technologies?
There’s the elements of history and the future to consider here. Research suggests that technology is not a threat to manufacturing jobs. Meanwhile in offices, technological progress has resulted in job displacement and higher skill requirements. With the digital transformation, a large number of jobs requiring a lower level of education have disappeared. These include assistant roles in the banking sector, which were sharply reduced when automation was introduced in the 1980s.
Scientists agree that it is very difficult to predict the future of work. There are significant uncertainties involved in forecasting the impact of artificial intelligence and novel digital technologies on the demand for specific skills and jobs.
Perhaps it might be helpful to think about artificial intelligence as a predictive technology, in other words using data to make better forecasts. Whose jobs will better forecasting improve and who are at risk of losing theirs? Most likely people in expert roles will benefit from AI, since easier access to high-quality data helps to make better decisions. Meanwhile, many jobs involving data collection and forecasting might be replaced with automation.
When you worked for Etla Economic Research, you were involved in the development of nowcasting. Can you elaborate on that?
We wanted to find out how we could improve economic forecasts by using big data. The word nowcasting refers to predicting the present as opposed to forecasting, which is concerned with what will happen in the future.
Usually, key social data is available with a significant delay. Compiling data such as unemployment statistics from surveys is a very time-consuming task. We built a forecasting model that uses real-time data collected by Google and data obtained from other sources.
Another very useful thing about big data is that it offers quantifiable information. Google’s search data tells us exactly how many people have applied for unemployment benefits online. And, in addition to current unemployment, search data also provides insights into future unemployment. People who know they will face unemployment long before their employment relationship is actually terminated often search for information online about things like unemployment benefits. These forecasts give us information on economic trends. For Finland and Europe, this method can generate a three-month outlook at least, but trends can be predicted as far ahead as six months.
Are there other applications for the big data-based forecasting method you described?
We can create forecasts at both the micro and the macro level. Etla built a macro model for housing prices, which studied online search behaviours to accurately predict housing price development in Finland. Other similar applications include predicting purchase decisions, or freight forecasting. Migration is another important area. Before the refugee crisis broke in 2015, people in war-stricken countries actively sought information on relocation, which indicated they were planning to leave their homes.
But at the micro level, big data offers even more interesting information. Specific information can be obtained on a certain company or product, or we can use data in Finnish registers such as the real-time incomes register, which tells us exactly how much Finnish people earn each month.